Tongxin Li
Assistant Professor, Presidential Young Fellow at CUHK-Shenzhen
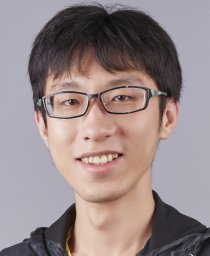
Teaching Complex Bldg, 608
School of Data Science
CUHK-Shenzhen
I am currently a tenure-track assistant professor in the School of Data Science (SDS) at The Chinese University of Hong Kong, Shenzhen (CUHK-Shenzhen).
Prior to joining SDS, I received a PhD in CMS at the California Institute of Technology. I interned twice as an applied scientist at AWS security in the summers of 2020 and 2021.
My research centers on interdisciplinary topics in control, online decision-making, optimization, and artificial intelligence (AI), and power systems. I am passionate about developing AI techniques that impact real-world physical complex systems. My previous work includes advancements in control with ML predictions and the integration of AI tools in smart grids.
Opening: I am looking for PhD students and Postdocs who are interested in contributing to the emerging domains of
-
Power systems
-
Control, reinforcement learning and online decision-making
Feel free to send me an email with your CV attached. Other formats of local and remote collaboration are also welcomed.